Introduction
In the ever-evolving landscape of finance and risk management, internal auditors are increasingly turning to data analytics to sharpen their risk-scoring techniques. It’s not just a trend; it’s a revolution. Did you know that organisations leveraging data analytics in their audit processes report a significant boost in risk detection accuracy(KPMG)? That’s not just a statistic; it’s a game-changer.
As someone who’s been in the trenches of internal audit for almost a decade, I’ve seen first-hand how data analytics has transformed internal audit. Remember the days of manual sampling and gut-feel risk assessments? They seem almost quaint now, don’t they? But let’s not get nostalgic – we’re here to talk about the future, and that future is data-driven.
In this comprehensive guide, we’ll dive deep into the world of internal audit risk scoring using data analytics. We’ll explore everything from the basics to advanced techniques, including impact-likelihood matrices and integrating insights from previous audits and the second line of defence information. Whether you’re a seasoned auditor looking to up your game or a data analyst venturing into the world of internal audit, this guide has something for you.
So, buckle up, fellow risk enthusiasts! We’re about to embark on a journey that will change your thoughts about audit risk scoring. Are you ready to turn data into your secret weapon? Let’s dive in!
Understanding the Basics of Internal Audit Risk Scoring
What’s the score?
Imagine you’re a detective, but instead of solving crimes, you’re uncovering potential risks in a company. That’s essentially what internal audit risk scoring is all about. It’s a systematic approach to evaluating and prioritising risks within an organisation. But why is it so crucial in modern auditing?
Let’s break it down:
- Definition: Internal audit risk scoring is the process of assigning numerical values to various risks based on their potential impact and likelihood of occurrence.
- Traditional vs. Data-Driven: Traditionally, risk scoring relied heavily on professional judgment and limited sampling. Today, data-driven approaches allow for a more comprehensive and objective assessment. It’s like upgrading from a magnifying glass to a high-powered microscope.
- Importance in Risk Management: Effective risk scoring is the backbone of risk management and mitigation strategies. It helps organisations focus their resources where they’re needed most.
The evolution of risk scoring
Remember when risk scoring was more art than science? We’d sit around a table, debating the likelihood of various scenarios like ancient Greek philosophers. While there’s still a place for professional judgment, data analytics has revolutionised the field.
Today, data-driven risk scoring is not just an enhancement—it’s a paradigm shift. By leveraging advanced analytics, organisations are better equipped to anticipate emerging risks, allowing for more proactive and informed decision-making.
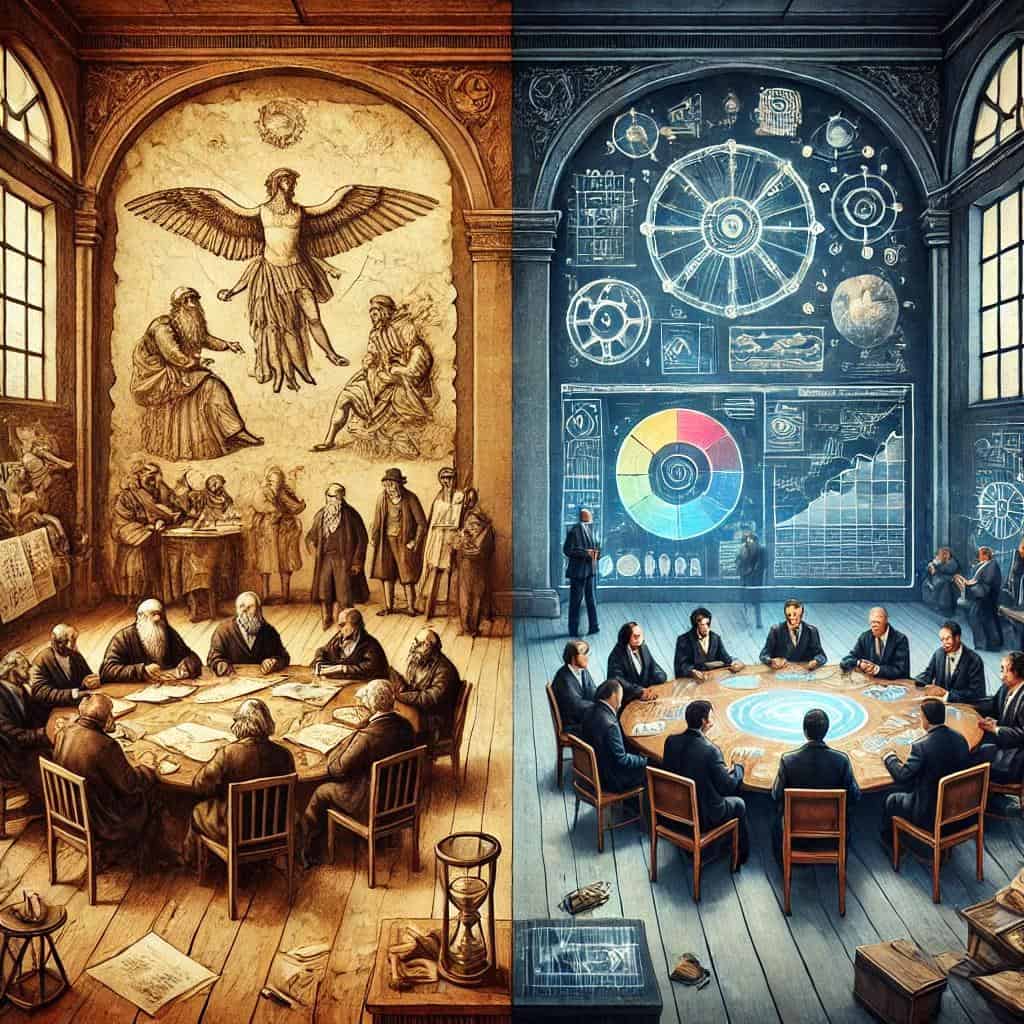
The Role of Data Analytics in Internal Audit
From gut feeling to data-driven decisions
Remember the last time you had a “hunch” about a particular risk? While intuition has its place, data analytics takes risk assessment to a whole new level. It’s like upgrading from a compass to GPS navigation.
Here’s how data analytics is transforming risk scoring processes:
- Enhanced Accuracy: By analysing vast amounts of data, we can identify patterns and anomalies that human eyes might miss.
- Improved Efficiency: Automated data analysis can process information faster than any human team, allowing for real-time risk assessments.
- Deeper Insights: Data analytics doesn’t just tell you what’s happening; it helps you understand why and what might happen next.
Key data analytics techniques in risk scoring
- Predictive Analytics: Using historical data to forecast future risks. It’s like having a crystal ball, but one based on actual data!
- Data Mining: Sifting through large datasets to uncover hidden patterns and relationships.
- Machine Learning: Algorithms that improve automatically through experience, continuously refining risk models.
Data Analytics Methods for Risk Scoring
The data analyst’s toolkit
As internal auditors, we’re no longer just number crunchers; we’re data scientists in disguise. Let’s dive into the key data analytics methods that are revolutionising risk scoring:
Predictive Analytics
- What it does: Uses historical data to predict future risks.
- How it helps: Imagine being able to forecast potential audit findings before you even start fieldwork. That’s the power of predictive analytics.
- Real-world example: A financial institution used predictive analytics to identify potential fraudulent transactions, reducing false positives by 60%.
Descriptive Analytics
- What it does: Analyses past data to understand risk trends and patterns.
- How it helps: It’s like having a time machine that lets you learn from past audit experiences to inform current risk assessments.
- Real-world example: A manufacturing company used descriptive analytics to identify recurring quality control issues, leading to a 25% reduction in defects.
Cluster Analysis
- What it does: Groups similar data points to identify potential risk areas.
- How it helps: It’s like sorting a jumbled box of Lego bricks into neat piles, making it easier to spot the odd pieces (risks) that don’t fit.
- Real-world example: A retail chain used cluster analysis to group stores by risk profile, allowing for more targeted audit approaches.
Regression Analysis
- What it does: Determines relationships between variables to assess risk factors.
- How it helps: It’s like being a detective, uncovering the hidden connections between different aspects of your organisation.
- Real-world example: An insurance company used regression analysis to identify key factors contributing to claim fraud, improving detection rates by 40%.
Anomaly Detection
- What it does: Identifies unusual patterns that could indicate potential risks.
- How it helps: Think of it as your organisation’s immune system, constantly on the lookout for anything out of the ordinary.
- Real-world example: A telecommunications company used anomaly detection to identify unusual network traffic patterns, preventing a major data breach.
Using Impact-Likelihood Risk Matrices with Data Analytics
The matrix reloaded
Impact-likelihood matrices have been a staple in risk assessment for years. But when supercharged with data analytics, they become a force to be reckoned with. Let’s break it down:
- Definition: An impact-likelihood matrix is a visual tool that plots risks based on their potential impact and probability of occurrence.
- The Data Analytics Twist: Instead of relying solely on subjective assessments, we now use data to populate and refine these matrices.
How data analytics enhances the matrix
Impact Assessment
- Traditional method: Educated guesses based on limited historical data.
- Data-driven approach: Using predictive models to quantify potential financial, operational, and reputational impacts.
Likelihood Assessment
- Traditional method: Subjective probability estimates.
- Data-driven approach: Leveraging historical data and trend analysis to calculate precise probabilities.
Continuous Monitoring
- Traditional method: Periodic updates to the matrix, often annually or quarterly.
- Data-driven approach: Real-time updates based on continuous data streams.
Exploring Alternative Risk Scoring Matrices
Beyond the traditional
While impact-likelihood matrices are powerful tools, they’re not the only game in town. Let’s explore some alternative risk scoring matrices that, when combined with data analytics, can provide even deeper insights:
1. Dynamic Heat Maps
- What They Are: Heat maps visually represent risk severity across different areas, using colour gradients to highlight risks. Unlike static matrices, dynamic heat maps update in real-time based on incoming data, offering a continuous and intuitive understanding of risk distribution.
- Internal Audit Perspective: By employing AI-driven dynamic heat maps, internal audit teams can monitor risks as they evolve. These maps automatically adjust to new data inputs, providing auditors with up-to-the-minute insights into risk concentrations and trends.
- Real-World Example: Investment banks have employed AI to enhance their market risk analysis through dynamic heat maps. Using AI algorithms to analyse large datasets, including social media trends and economic indicators, these heat maps can predict market volatility and highlight areas of potential risk. Traders can adjust their strategies in real-time, reducing the likelihood of significant losses
2. Enhanced Risk Control Matrices (RCM)
- What It Is: A Risk Control Matrix (RCM) is a structured tool documenting the relationship between risks, controls, and their effectiveness. It ensures that identified risks are adequately managed by implementing specific controls.
- Internal Audit Perspective: Internal audit teams can enhance the traditional RCM by integrating continuous auditing and AI-based automated control testing. This approach allows for real-time validation of controls, ensuring they remain effective over time without the need for periodic manual reviews.
- Real-World Example: JPMorgan Chase’s use of the COiN (Contract Intelligence) system exemplifies this approach. COiN uses machine learning to review and interpret commercial loan agreements, which once required thousands of hours of manual review. By automating this process, JPMorgan Chase has dramatically improved the accuracy and efficiency of their risk assessments, ensuring that all contractual risks are effectively managed(IRM India).
3. Data-Driven Bowtie Analysis
- What It Is: Bowtie analysis is a graphical tool that maps the pathways from potential risk events to their consequences, along with preventive and mitigating controls. It provides a comprehensive view of risk management, from causes to impacts.
- Internal Audit Perspective: By incorporating historical data and predictive analytics, internal audit teams can quantify the effectiveness of different controls within the bowtie framework. This data-driven approach prioritises controls based on their proven effectiveness in mitigating risks.
- Real-World Example: Wells Fargo implemented an AI-driven fraud detection system that aligns with the principles of bowtie analysis. The system analyzes transaction patterns in real-time to detect and prevent fraud. By continuously learning from new data, the system adapts to emerging threats, ensuring that preventive controls remain practical and up-to-date
4. Risk Exposure Matrices
- What They Are: Risk exposure matrices assess potential loss by considering the likelihood of a risk event and the level of exposure an organisation has to that risk. This approach focuses on the specific vulnerabilities and potential financial impacts.
- Internal Audit Perspective: Internal audit teams can enhance risk exposure matrices by incorporating real-time market data and predictive models. This allows for more accurate assessments of potential financial or operational losses, enabling dynamic adjustments to risk management strategies.
- Real-World Example: Santander uses AI-powered predictive analytics to monitor its loan portfolio. The system continuously updates risk exposure matrices by analyzing account behaviours and economic trends. This allows Santander to proactively manage risks by identifying at-risk customers early and offering them tailored support, thereby reducing the bank’s exposure to loan defaults.
The power of integration
The real magic happens when we integrate these matrices with data analytics. By combining advanced analytics with traditional tools, organisations can achieve greater precision in risk prediction and prevention, ultimately enhancing their overall risk management strategies.
But here’s a question to consider: With so many options available, how do you choose the right matrix for your organisation? The answer lies in understanding your specific risk landscape and data capabilities.
Leveraging Previous Audit and Assurance Outcomes in Risk Scoring
Learning from the past
As the saying goes, “Those who cannot remember the past are condemned to repeat it.” In the world of internal audit, this couldn’t be more true. But how do we effectively leverage insights from previous audits to enhance our current risk scoring? Let’s dive in:
Historical Data Analysis
- What it is: Using past audit outcomes to predict future risks and adjust risk scores accordingly.
- How it helps: It’s like having a time machine that allows you to learn from past experiences to inform current decisions.
Outcome Correlation
- What it is: Correlating audit findings with risk factors to enhance the accuracy of risk scoring models.
- How it helps: It’s like connecting the dots between seemingly unrelated events to uncover hidden risk patterns.
Trend Analysis
- What it is: Identifying patterns and trends in audit outcomes over time.
- How it helps: It’s like having a crystal ball that shows you where your organisation’s risk landscape is heading.
Integrating Insights from 2nd Line, 1B, and Data Analytics for Enhanced Risk Scoring
The power of collaboration
In the world of risk management, silos are our enemy. By integrating insights from various lines of defence, we can create a more holistic and accurate risk scoring model. Let’s break it down:
Second Line of Defense Insights
- What it is: Information from risk management and compliance functions.
- How it helps: It provides a broader perspective on organisational risks and control effectiveness.
1B Insights
- What it is: Inputs from specialised control functions, such as legal and finance.
- How it helps: Offers a granular view of risk in specific areas, contributing to a more nuanced risk assessment.
Data Analytics
- What it does: Automates the process of combining and analysing insights from different lines of defence.
But here’s a challenge: How do you break down silos and ensure that your entire organisation benefits from integrated risk scoring? The key lies in fostering a culture of collaboration and transparency.
How Risk Scoring is Applied Across the Organisation
Where does this fit?
Risk scoring isn’t a one-size-fits-all approach. It needs to be tailored to different levels of the organisation.
Entity-Level Risk Scoring
- The big picture: Entity-level risk scoring assesses risks that impact the organisation, including strategic, financial, operational, and reputational risks.
- Why is it crucial?: At this level, risk scoring aligns with organisational objectives, mission, and vision. Multinational corporations consider global risks, such as geopolitical risks and cross-border regulatory compliance.
Business Unit/Department-Level Risk Scoring
- Zooming in: Business unit or department-level risk scoring evaluates risks specific to individual departments, such as finance, operations, HR, and IT.
- What’s the focus?: Integrating department-specific key risk indicators (KRIs) into the risk scoring model is essential. Collaboration between departments ensures accurate risk scoring, particularly in highly regulated sectors like healthcare, finance, and energy.
Process-Level Risk Scoring
- Getting granular: Process-level risk scoring focuses on specific processes within departments that may carry significant risks, such as supply chain management or financial reporting.
- Why does it matter?: Incorporating data from process controls and performance metrics enhances risk scoring accuracy. Continuous process monitoring and automation play a critical role in improving risk scoring at this level.
Project-Level Risk Scoring
- Project by project: Project-level risk scoring addresses risks associated with specific projects, such as new product launches or mergers and acquisitions.
- How to handle this?: Applying risk scoring to project management involves evaluating factors like timelines, resource allocation, and potential impact on other business areas. Scenario analysis and data modelling are key tools for predicting project risks.
Subsidiary/Regional-Level Risk Scoring
- Geographical focus: Subsidiary or regional-level risk scoring evaluates risks unique to subsidiaries or regional operations, particularly in large, geographically dispersed organisations.
- Why focus here?: This level of risk scoring addresses local regulatory compliance risks, market-specific risks, and cultural considerations, integrating these scores into the broader enterprise risk management (ERM) framework.
Compliance and Regulatory Risk Scoring
- Staying compliant: Compliance and regulatory risk scoring assesses risks related to adherence to industry-specific regulations, such as GDPR, SOX, HIPAA, or environmental regulations.
- Why is this critical?: Risk scoring in this context helps maintain regulatory compliance and avoid penalties. Data analytics can be used to monitor compliance risks in real-time, providing early warnings and mitigating actions.
Third-Party and Supply Chain Risk Scoring
- Looking outward: Third-party and supply chain risk scoring evaluates risks associated with external vendors, suppliers, and partners.
- What’s the strategy?: Incorporating data from supplier audits, performance reviews, and market trends into the risk scoring model is essential. This approach helps mitigate the impact of supply chain disruptions on organisational risk.
Technology and Cybersecurity Risk Scoring
- Protecting the digital realm: Technology and cybersecurity risk scoring focuses on risks related to IT systems, cybersecurity, data breaches, and technology disruptions.
- Why is this a priority?: With the increasing threat of cyber-attacks, data analytics plays a vital role in monitoring and assessing cybersecurity threats in real-time. Regular cybersecurity audits should be integrated into the risk scoring process.
Environmental, Social, and Governance (ESG) Risk Scoring
- Embracing responsibility: ESG risk scoring evaluates risks related to environmental impact, social responsibility, and corporate governance.
- Why is it trending?: ESG factors are increasingly becoming a critical component of risk scoring, especially for companies facing investor scrutiny. Integrating ESG data and metrics into the overall risk scoring model is essential for long-term sustainability.
Industry-Specific Risk Scoring
- Tailoring the approach: Industry-specific risk scoring addresses risks unique to certain industries, such as financial services, healthcare, manufacturing, or technology.
- What’s the benefit?: Customising risk scoring frameworks to account for industry-specific regulations, market conditions, and operational challenges ensures more accurate and relevant assessments.
Crisis and Business Continuity Risk Scoring
- Preparing for the worst: Crisis and business continuity risk scoring assesses risks related to potential crises, such as natural disasters, pandemics, or major operational failures.
- How to be ready?: Incorporating business continuity planning into the risk scoring process is crucial. Data analytics can simulate crisis scenarios, helping evaluate the organisation’s preparedness and resilience.
Overcoming Challenges in Data-Driven Risk Scoring
Is it all smooth sailing?
Not quite. Adopting data analytics for risk scoring comes with its challenges.
Common Obstacles
Facing reality
Auditors often encounter challenges such as data quality issues, lack of data integration, and resistance to change.
Practical Solutions
Getting it right
To overcome these obstacles, it’s essential to focus on data quality, invest in the right tools, and foster a culture of continuous learning and upskilling in data analytics.
Why persevere?
While the journey may be challenging, the rewards—more accurate risk assessments and better decision-making—are well worth the effort.
Best Practices for Enhancing Risk Scoring with Data Analytics
Ready to level up?
Here’s how you can enhance your risk scoring processes with data analytics.
Industry Best Practices
What works?
Integrating data analytics into audit risk scoring requires continuous monitoring and updating of risk models. Collaboration between audit teams and data specialists is also crucial.
Continuous Monitoring
Staying ahead
The key to effective risk scoring is continuous monitoring. By regularly updating your models with the latest data, you can ensure that your assessments remain accurate and relevant.
Collaboration is Key
Why go it alone?
Collaboration between audit teams and data specialists is essential for success. By working together, you can leverage the strengths of both groups to create a more effective risk scoring process.
Conclusion: Embracing Data-Driven Risk Scoring in 2024
The integration of data analytics into internal audit risk scoring is not just a trend—it’s the future. By embracing these techniques, auditors can enhance their processes, improve accuracy, and stay ahead in the rapidly evolving financial landscape.
Curious about how you can supercharge your audits with AI? Discover the secrets in my ultimate guide to using AI in internal audit and risk management—you won’t want to miss this game-changer!
Are you ready to take your auditing to the next level? Don’t hesitate. Start incorporating data-driven methods into your risk scoring today, and watch your audit effectiveness soar.
Let’s keep the conversation going!
What’s your biggest challenge when integrating data analytics into your audit processes? Comment below—I’d love to hear your thoughts! And if you’re keen on staying updated with the latest in Data Science and AI in Internal Audit, don’t forget to join my newsletter.